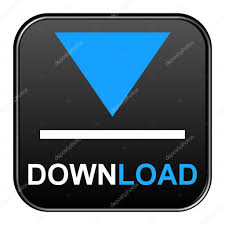
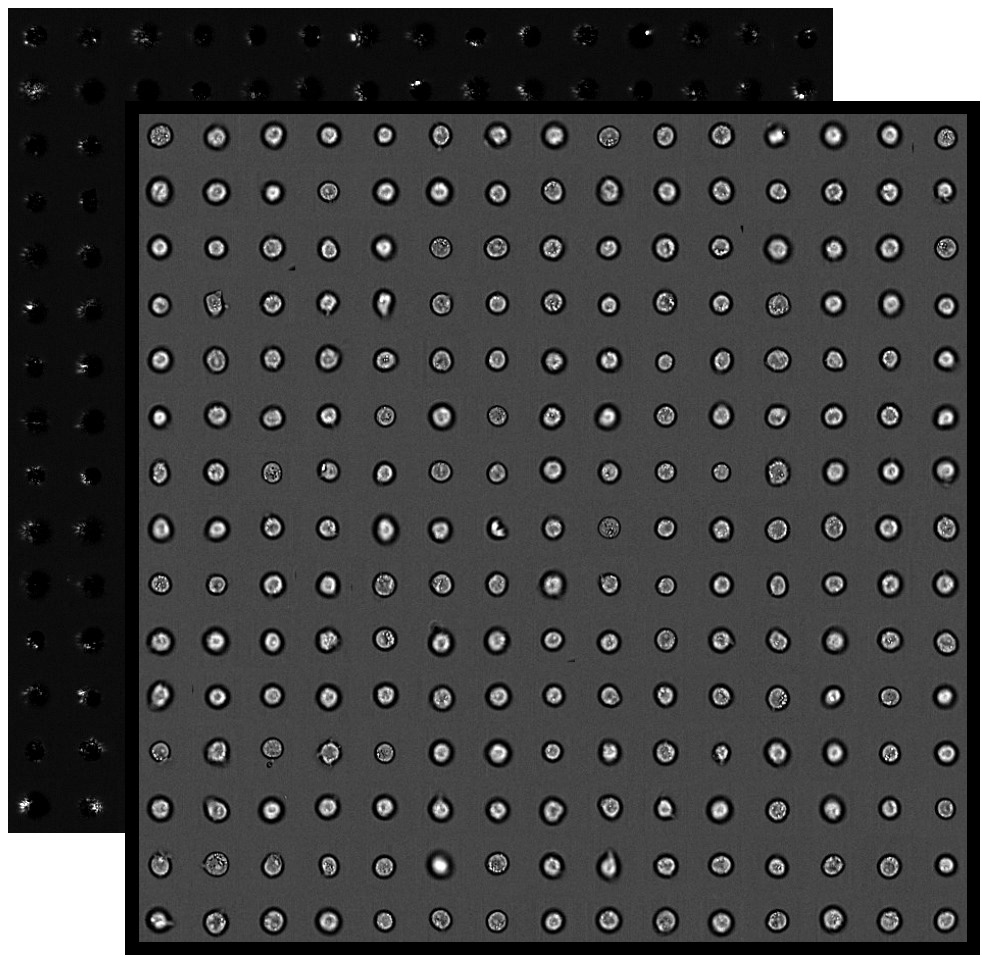
However, fluorescence cell imaging has significant drawbacks. One key advantage is that multiple fluorescence images of the same cell culture can be acquired in parallel using the appropriate combination of chemical dyes and light filters, with the resulting images containing information about different cell structures. 1(a): the cell structures of interest are chemically stained using fluorescence staining techniques fluorescence images are acquired and, finally, these images are analyzed to retrieve quantitative measures about the cell structures of interest. Kepp,Ĭell death assays for drug discovery,” Nat. and to determine the uptake and toxicity of drugs. Identification of pathways regulating cell size and cell-cycle progression by RNAi,” Nature 439, 1009– 1013 (2006). Rizzuto,Ĭhimeric green fluorescent protein as a tool for visualizing subcellular organelles in living cells,” Curr. For example, changes in the morphological properties of cell structures are used to monitor the physiological state of a cell culture, 1 1.
CELLPROFILER COUNT AGGREGATES AROUND NUCLEUS SOFTWARE
To make this deep-learning–powered approach readily available for other users, we provide a Python software package, which can be easily personalized and optimized for specific virtual-staining and cell-profiling applications.īiomedical and pharmaceutical research often relies on the quantitative analysis of cell structures. Generating virtually stained fluorescence images is less invasive, less expensive, and more reproducible than standard chemical staining furthermore, it frees up the fluorescence microscopy channels for other analytical probes, thus increasing the amount of information that can be extracted from each cell. Subsequently, we use these virtually stained images to extract quantitative measures about these cell structures. Specifically, we train a cGAN to virtually stain lipid droplets, cytoplasm, and nuclei using bright-field images of human stem-cell–derived fat cells (adipocytes), which are of particular interest for nanomedicine and vaccine development. We show that this is a robust and fast-converging approach to generate virtually stained images from the bright-field images and, in subsequent downstream analyses, to quantify the properties of cell structures. Here, we introduce an alternative deep-learning–powered approach based on the analysis of bright-field images by a conditional generative adversarial neural network (cGAN). However, these techniques are often invasive and sometimes even toxic to the cells, in addition to being time consuming, labor intensive, and expensive.
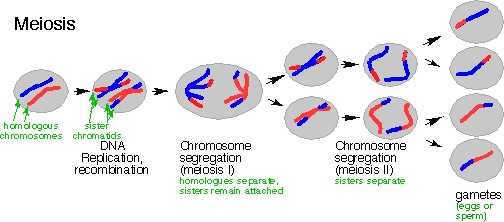
The standard imaging approach relies on fluorescence microscopy, where cell structures of interest are labeled by chemical staining techniques. Quantitative analysis of cell structures is essential for biomedical and pharmaceutical research.
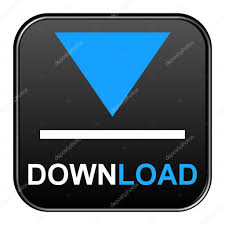